Keynote Speakers
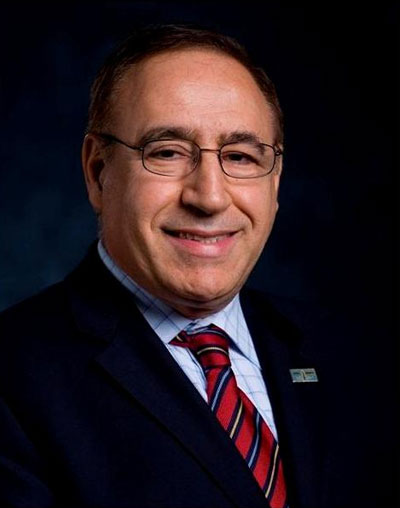
Metin Akay
Founding Chair, John S Dunn Endowed Chair Professor, University of Houston
Brain Cancer Chip for Precision Medicine
Glioblastoma multiforma (GBM) is the most common and malignant primary brain tumor in adults because of its highly invasive behavior. The best existing treatment for GBM involves a combination of resection, chemotherapy and radiotherapy, and has a very limited success rate with a median survival rate of less than 1 year. Therefore, there is an urgent need to reduce the time of the preclinical brain tumor growth studies by developing large-scale in vitro cancer platforms for cost-effective high-throughput screening of novel cancer drugs and therapeutics and assessment of treatment responses.
The aim of our project is to develop a novel 3D brain cancer chip as a tool for high-throughput drug screening in order to determine personalized treatment plans for individual brain cancer patients in a matter of weeks. The brain cancer chip we have developed is composed of photopolymerizable poly(ethylene) glycol diacrylate (PEGDA) hydrogel, and integrates a microwell array with microfluidic channels. This chip allows for the simultaneous administration of up to three drugs while establishing a concentration gradient that supplies a unique mixture of drugs to each microwell. Therefore, our brain cancer chip provides a sustainable, high-throughput 3D tissue formation platform for multi-drug testing. Our preliminary studies strongly encourage us to utilize the brain cancer chip to determine the optimal combination of anticancer drugs, using only a tiny tissue sample collected from a patient by biopsy, to most effectively treat GBM in patients in a shorter period of time.
We believe that the chip will also be useful and cost-effective for high-throughput screening of cancer drugs and assessment of treatment responses, and could pave the way for precision medicine in cancer treatment.
In collaboration with Drs. H Xia, N Avci, YM Akay
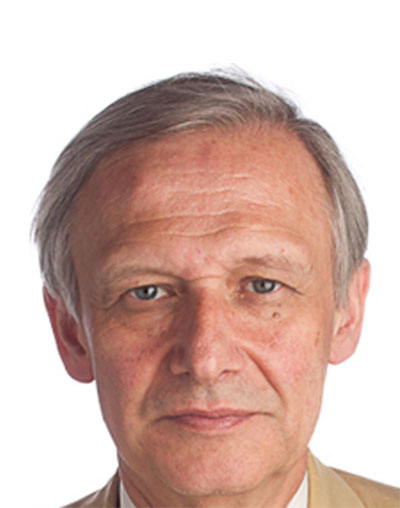
Sergio Cerutti
Department of Electronics, Information and Bioengineering (DEIB), B-cube Laboratory: Biosignals, Bioimages and Bioinformatics, Politecnico di Milano, Italy
Advances in Biomedical Signal Processing: Multivariate, Multimodal, Multiorgan and Multiscale Integration of Information for Precision Medicine and Big Data Analysis
The lecture will focus on advanced concepts of biomedical signals and data processing which are intended as a target to be reached towards the fulfillment and implementation of original paradigms for new insights into Physiology and Medicine. As widely demonstrated in the literature, the so-called 4M-paradigm (Multivariate, Multiorgan, Multimodal, and Multiscale) allows to obtain a remarkable fusion of information which is able to produce a significant added value in respect to single signal-based approach, and the development of new algorithms, new technologies, and new global bioinformatic systems facilitates such an integration process, where our Discipline of Biomedical Engineering has always had a pivotal leading role. Along this research approach, two apparent conflicting concepts like Precision Medicine (to access to precious biomarkers relevant to each single patient) and Big Data Analysis (to access to a huge number of data of “homogeneous” large cohort of patients) might converge to creating a formidable tool for feature selections from signals and data, for diagnostic classification, for therapy evaluation and patient’s follow-up. I am wondering how powerful could result in an investigation in which the “intelligence” of a model-driven approach (including models, but also data, biosignals, and bioimages) is combined with the “powerfulness” of a data-driven approach which employs sophisticated algorithms of big data analysis. This is an opportunity, rather than a threat, and I wish to launch this message to the wide and always increasing community of biomedical engineers who will have to cope in the future with this intriguing and fascinating information process.
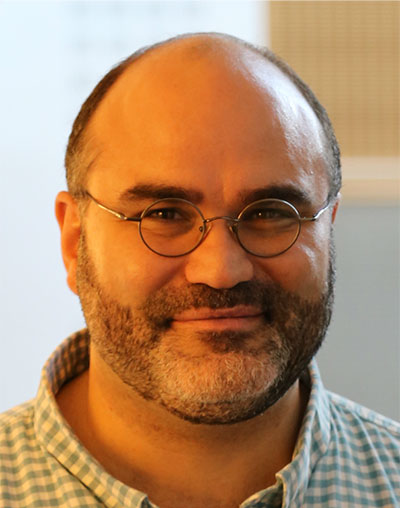
Alejandro F. Frangi
Center for Computational Imaging & Simulation Technologies in Biomedicine, University of Leeds, UK
Computational Medicine: from advanced diagnosis and interventional planning to in silico trials of endovascular devices
Computational Medicine (CompMed) is an emerging discipline devoted to developing quantitative approaches for understanding the mechanisms, diagnoses, and treatment of human disease through the systematic application of mathematics, engineering, and computational science. Dealing with the extraordinary multi-scale complexity and variability intrinsic to human biological systems and health data demands radically new approaches compared to methods for manufactured systems.
Using intracranial cerebral aneurysms as clinical driving problem, this keynote will focus on and illustrate two specific aspects: a) how the integration of biomedical imaging and sensing, signal and image computing and computational physiology are essential components in addressing this personalized, predictive and integrative healthcare challenge, and b) how such principles could be put at work to support clinical decision and inform medical device trials.
Finally, this keynote will also underline the important role of model validation as a key to translational success and how such validations span from technical validation of specific modeling components to clinical assessment of the effectiveness of the proposed tools. To conclude, the talk will outline some areas where current research efforts fall short in Computational Medicine and that might benefit from further investigation in the upcoming years.
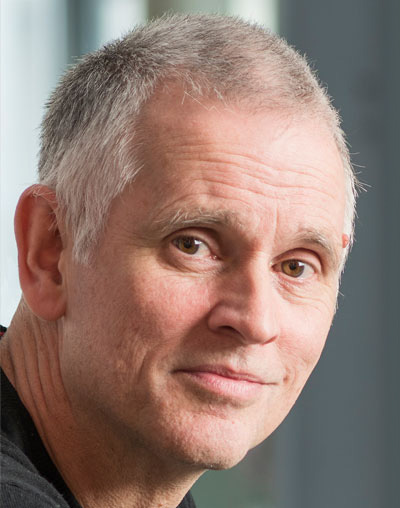
Dave Robertson
Head of College of Science & Engineering, The University of Edinburgh, Edinburgh, Scotland, UK
Building a Knowledge Graph for UK Health Data Science
Digital healthcare offers the prospect of being able to generate and combine diverse data at large scale across the health system and use this to produce knowledge which is actionable for clinical care. Precision medicine (because it focuses narrowly on specific sets of attributes in order to target treatments) drives data sets and cohort sizes to be large and extensive across populations. To achieve this scale requires access to a wide variety of data sets (primary, secondary, social care, etc) across many dimensions (genotypic, phenotypic, etc). These data sets typically are curated locally but the analyses we need to perform on them will apply across local jurisdictions, so the data set needed for a typical analysis (or experiment in the case of research) must be formed from the appropriate subsets of local data sets and assembled in a secure, federated architecture. To federate data for analysis at this scale we require standardisation of access to local data sets via a shared ontology (i.e. a formal system of description across the local data schemas) that provides a “map” of the available data. Marshalling of the data for analysis is then achieved by specifying (at the level of the ontology) the query that describes the required data, with the federation system assembling the data across local data sets to generate the required data set for that specific analysis. This fully automates what is currently a time consuming and error prone process, making it possible to derive new knowledge much more rapidly than hitherto. This knowledge can then be used to influence clinical care; enrich local data sets (through feedback on consistency, etc) and generate healthcare knowledge that would be inaccessible from local data curators working alone.
This is easy to explain but difficult, in practice, to do. I will describe our work with a graph-based (i.e. relational network) data representation to define an interlingua that connects participating data sources via a core ontology that describes (through terminology mapping) how data may be linked. We have demonstrated, for a representative selection of data sources in Scotland, how they may be linked via their data schemas and how queries (and query formation) may be automated. This provides an executable specification of the “map” of data, independent of the data sets themselves, that can be used to open up a constellation of new services around the emerging Health Data Research UK data federation consortium.
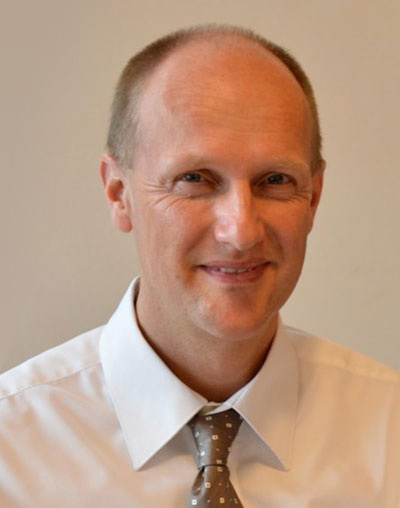
Carsten H. Wolters
Institute for Biomagnetism and Biosignal analysis, University of Münster, Germany
Reconstruction and manipulation of neuronal networks in the human brain
In my talk, I will present new methods and applications for multimodal brain imaging and brain stimulation to reconstruct and manipulate neuronal networks in the human brain. Brain imaging methods include modalities such as Electroencephalography (EEG), Magnetoencephalography (MEG), Magnetic Resonance Imaging (MRI) and diffusion MRI (dMRI). A special focus will be on the development of multimodal imaging and combined EEG/MEG/MRI source reconstruction methods using new forward and inverse approaches. In the brain stimulation research field I will discuss new optimization methods for multi-sensor setups to transcranial electric (TES) and magnetic stimulation (TMS) and combined TES/TMS. My talk will also include new artifact-correction, linear and non-linear registration as well as segmentation approaches for structural MRI such as T1-MRI, T2-MRI, and dMRI. I will show, how the new methodology can be applied in the field of neuroscientific brain research and in clinical applications such as presurgical epilepsy diagnosis.